On January 10, 1901, the Spindletop oil gusher erupted near Beaumont, Texas, propelling oil over 150 feet into the air at a rate of approximately 100,000 barrels per day. This marked the dawn of the modern petroleum industry, a systematic harvesting of a latent resource (oil) unlocked by the fevered intersection of technology (rigs, refineries, pipelines, engines, cars etc) and speculation.
The Spindletop oil gusher launches the gasoline era in 1901
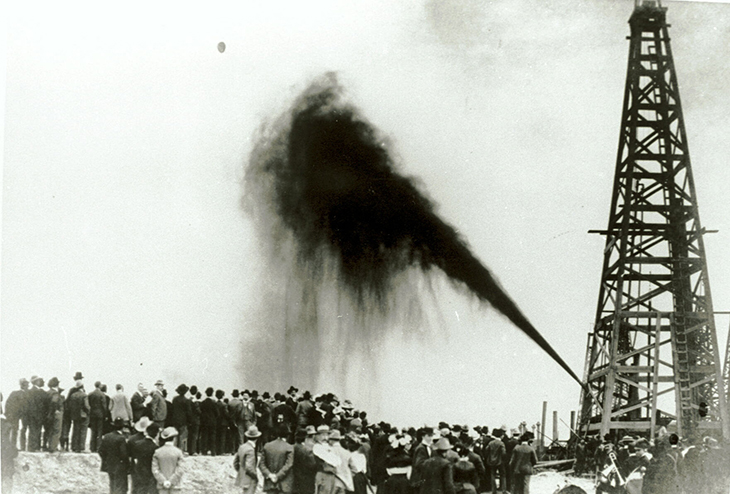
On November 30, 2022, OpenAI unveiled ChatGPT, a conversational AI model trained ~570 GB of internet text data that gained over 100 million users within two months. This marked the dawn of the modern AI era, a systematic harvesting of a latent resource (data) unlocked by the fevered intersection of technology (data centers, databases, networks, models, interfaces, etc) and speculation.
OpenAI Dev Day 2023
Like oil fields, there are a limited number of big data resources. As the co-founder and former Chief Scientist of OpenAI, Ilya Sutskever, recently said at the 2024 NeurIPS conference, “We have but one internet.” The question is just how limited are our data resources? Will they be the bottleneck for scaling laws? Are there still data gushers out there?
“We have but one internet” aka the Fossil Fuel AI - Ilya Sutskever
Through the early 20th century, independent oil prospectors known as “wildcatters” took massive financial risks in hopes of finding oil in unproven territories without established reserves … of hitting their own gusher. Many did. In 1912, Thomas B. Slick Sr. drilled the Wheeler No. 1 well, which became the discovery well for the prolific Drumright-Cushing oilfield, producing for the next 35 years. In the seven years after discovery (good venture investing timeline), the field had produced approximately 236 million barrels of oil or ~$5-6B of revenue in 2024 dollars.
Like the wildcatters, we believe that there are data gushers — information that is currently screaming into the abyss just waiting to be sensed and parsed — still out there. In fact, we believe AI has created a reflexive environment (see our essay on reflexivity), where advancements unlock new data sources which improve AI, which unlocks new data sources ad infinitum. In the rest of this essay, we will lay out how to find companies creating and capturing data gushers, which of those companies we think we are best suited to invest in, and what problems remain to be solved to release as many data gushers as possible.
How to Find a Data Gusher
Companies using data to produce value have always existed at the intersection of improvements in sensors and analytics. However, data gushers emerge when breakthroughs in sensing or analytics open up vast new fields of opportunity. For example, by reducing the cost of payload by ~20x, SpaceX has driven breakout improvement in satellite based sensing techniques, unlocking data gushers in industries like mining (see Fleet Space, Ideon, or Lunasonde). Thanks to generative pre-trained transformers (GPTs), analytics is going through a period of breakout performance improvement, unlocking a new field of potential data gushers for daring prospectors (more on this to come).
Data Gushers at the Intersection of Analytics & Sensor Improvements
Better sensors expand what is capturable at low cost. To invest in opportunities emerging from these improvements requires either extrapolating learning curves (eg. camera resolution vs cost) or predicting the applications of new techniques (eg. Osmo [where Geoffrey Hinton just joined the advisory board] enabling computers to smell). We believe multi-model sensing approaches (vision + hearing + smell) will see significant applications in industrial settings that require high bars of quality control and safety.
Analysis Improves Along Two Axes: Context & Content
Better analytics expands what is legible at low cost. Analytics can improve along two axes, context (what does it mean) and content (what is happening). Although machine learning has been a driver of analytical advancement, until GPTs it has largely been relegated to improvements along the content axis → regressions to predict energy demand, clustering for anomaly detection, etc. GPTs can now understand abstract concepts (business strategies or goals) that give meaning (context) to data streams at ever increasing scales (eg. larger context windows for retrieval augmented generation). To invest in opportunities emerging from these improvements requires either extrapolating scaling laws (eg. feasibility of 1M GPU datacenter coherence) or predicting the applications of new techniques (eg. Genesis Project a comprehensive physics simulation platform designed for general purpose robotics). These techniques can result in unintuitive outcomes, like WiFi being used to track body positions as demonstrated at Carnegie Mellon with DensePose.
Tracking Body Position in Rooms with WiFi
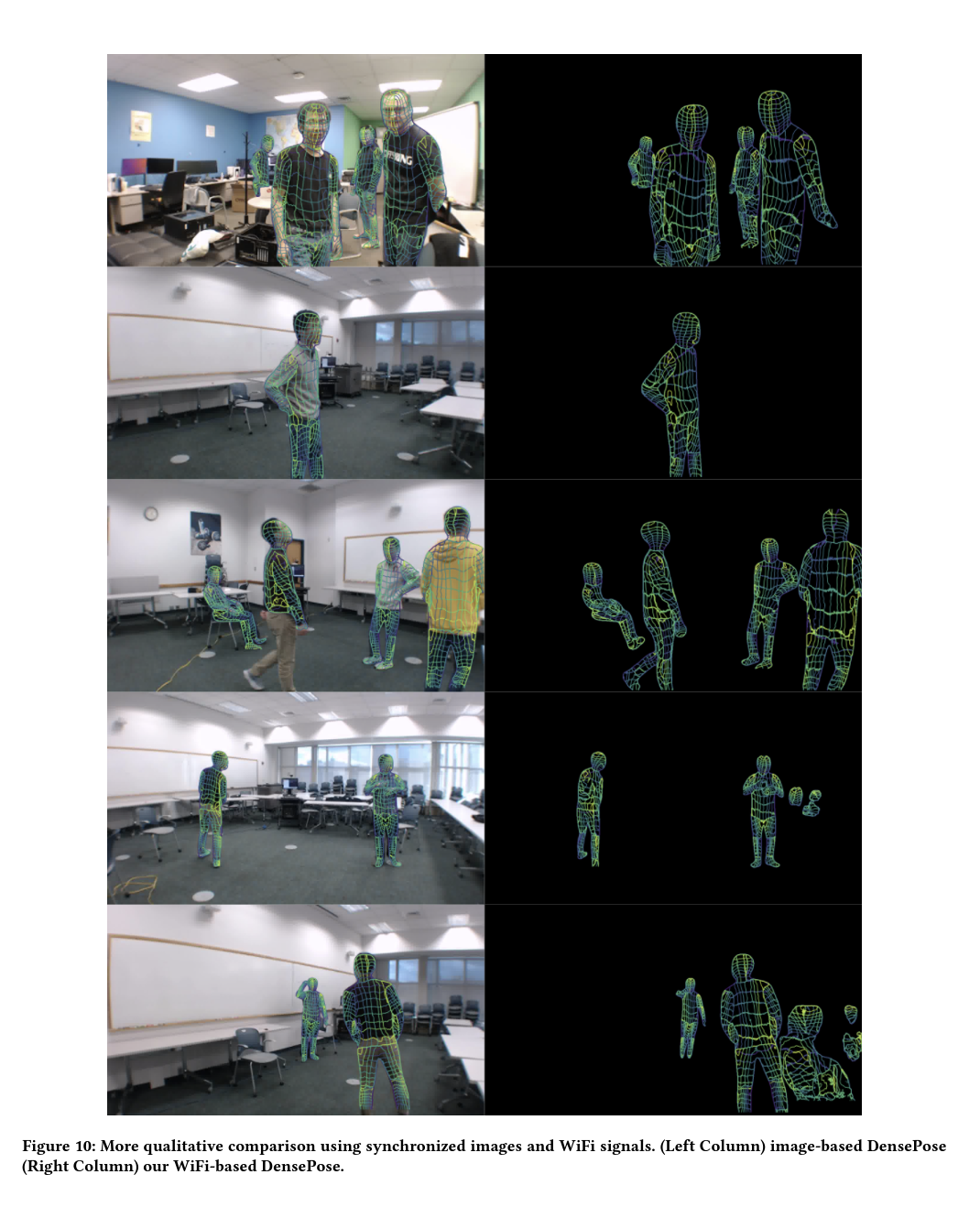
Incremental improvements in sensors and analytics are occurring all the time. However, we propose that when breakout improvements occur in either, data gushers emerge. When captured by companies, the products enabled by these data gushers have fast adoption due to the often visceral sense of relief (“finally we can know X easily”) or realization (“we had no idea Y was possible”) they deliver. These companies fall into two categories, the low hanging fruit and magical fruit. Both can make great investments but each favors a different type of VC.
Low Hanging Fruit
The low hanging fruit are companies that capture new data gushers by taking advantage of a single axis of breakout improvement (sensor xor analytics). Given the current wave of AI enabled analytics improvement, we are primarily seeing low hanging fruit companies deriving new data streams from existing sensing infrastructure.
Strayos Sensor Integrations for Mining
The success of these companies is determined by their ability to either develop methods of accessing sensor data (eg. Granola’s capture virtual meetings without joining a meeting) or simply doing the tedious integration work that others don’t want to do (eg. Strayos is providing real-time mine performance insights via years of building integrations with drone, satellite, and equipment sensing systems). When customers discover these products that quickly plug into existing infrastructure without downtime or disrupting workflows, the overwhelming refrain is one of relief.
Granola Delivering Meeting Notes “Relief”
The modern factory floor exemplifies a critical data integration challenge that impedes AI adoption in manufacturing. While automated machinery continuously generates vast amounts of sensor data, process logs, and operational metrics, this information remains trapped in isolated silos — stored locally in individual machines or segregated systems. This fragmentation prevents manufacturers from building the comprehensive datasets needed for effective AI implementation, despite factories theoretically generating more than enough data for advanced analytics.
Though statistical process control has used manufacturing data for decades, only a small fraction of available information gets actively utilized for optimization, while potentially valuable "data exhaust" goes unused because companies lack the infrastructure and expertise to extract meaningful insights from it. This disconnect between data generation and data utilization represents a significant barrier to Industry 4.0 initiatives and highlights the need for better data integration solutions in manufacturing.
What the modern factory operator knows is that they could understand more about their performance. The VC knows they could take better notes. But, neither wants to change how they work to do so. The best low hanging fruit solves this. However, given that these companies take often take advantage of breakthroughs that are available to the market as whole (eg. API access to frontier LLMs), copy-cat competition will likely be significant. For example, although Granola is breaking out, many companies offer similar solutions (Claap, Krisp, Parrot, Circleback, Shadow, etc).
Thus, once product market fit is achieved, these companies benefit from pure-play growth financing to drive customer acquisition and achieve escape velocity. For this reason, targeting low hanging fruit is best suited for large multi-stage firms that can more easily track the market as a whole via large outbound sourcing teams and deploy multiple tranches of capital to outspend the competition on customer acquisition if needed. The result of this is that the valuations for low hanging fruit companies tend to get bid up early in their lifecycle only to be compressed if multiple late stage players emerge, ultimately compromising returns.
Magical Fruit
The magical fruit are companies that capture new data gushers by taking advantage of both axes (sensor and analytics) including at least one of which has breakout improvement (sensor or analytics). In the current wave of breakout AI improvements, the success of companies in this category is determined by their ability to develop and deploy new sensing systems at scale that address known gaps in the industries they target.
For example, there is a known problem in the satellite industry of both man-made and natural space debris destroying satellites. Not only is this a significant problem on a per satellite basis due to their high cost, it has the catastrophic potential that as more satellites enter orbit the likelihood of a cascading set of collisions occurring that makes space inaccessible (known as Kessler Syndrome) increases. Talk about volatility! One way to solve this problem is tracking all the debris so that it can be avoided. Unfortunately today’s visual sensing systems have a data gap in the sub-centimeter diameter spectrum. That may seem insignificant, but a one centimeter diameter piece of debris traveling at 7.5 km per second has the energy equal to a hand grenade.
Data Gusher Opportunity in Space Debris
If a company could develop methods for identifying and tracking sub-centimeter debris using novel sensors (non-visual?) techniques with scalable analytical methods, they would unlock a data gusher that would have the potential to revolutionize the satellite market, in particular its lack of data-driven satellite insurance products. Beyond developing the sensor and analytical methods, this type of space debris company, like other potential magical fruits, would need a way to deploy these sensors at scale to unlock the full value of their solution for the market (eg. mapping all space debris across orbital lanes). Luckily, venture capital is perfectly suited for that.
Unlike for low-hanging fruit, we believe these companies combining sensor and analytical advancements are most likely to be identified early by thesis-driven early stage funds. This is because these firms are capable of both identifying existing data gaps in their areas of focus and evaluating the feasibility / capabilities of the sensor technology in particular. Once technically underwritten, the VC can fund the upfront deployment prior to revenue generation that both unlocks customers and gives the company a scale advantage (and potentially network effect) that prevents future competition.
GenLogs Delivering “Realizations” to the Freight Market
At Steel Atlas, our lead check in GenLogs’ Seed Round was a perfect example of this. GenLogs developed their own sensor system (combining multi-factor visual and radio frequency methods) for tracking freight across the US highway system. GenLogs believed that if deployed at sufficient scale, they could use their sensor network to track nearly 100% of US freight in real time to defeat fraud, stop human trafficking, and dramatically improve freight market liquidity, a $100B+ opportunity. Given our depth in the space (including past personal investments in companies like Flexport and Project44), we were able to underwrite those claims where other more generalist multi-stage firms were not. This fall, GenLogs released their product and, in classic data gusher fashion, hit hockey stick growth converting and producing "revelatory" customer reactions — “how did we even do this before X?”.
Gecko Robotics Novel Sensing Robot for Critical Infrastructure
Whether it is Anduril’s original Sentry product for persistent autonomous awareness across land, sea and air (now combined with their Lattice SDK - more on Lattice from Ben Thompson) or Gecko Robotics solutions for fixed sensors and robots that climb, crawl, swim, and fly to produce data on critical infrastructure analyzed by their Cantilever system — these companies are often category defining. Furthermore because of the level of the novelty of the data they gather and analyze, these companies are uniquely positioned to either operate as or facilitate the underwriting of insurance products. We view this as the fast path to becoming an entrenched incumbent that we are excited to support in our investments to come. As Brad Jacobs might say, we think this is a good way to make a few billion dollars.
How Palantir Became Worth ~$175B+ Overnight
Palantir Quarterly Revenue & Market Cap
Palantir was a good company for a long time (as of 12/20/23 its EV / NTM revenue was 13.9x). This year though, the market decided Palantir is a great company (as of 12/20/24 its EV / NTM revenue was 49.7x). What happened? It became a data gusher “overnight.” As LLMs made the breakout transition from feasible in enterprise / government applications (2023) to functional (2024), Palantir’s integration work was re-valued.
History may not repeat, but it definitely rhymes. Palantir’s playbook parallels Standard Oil’s. Instead of focusing on the acquisition of undervalued land rights (oil prospecting) and deploying drilling rigs, under John D. Rockefeller’s leadership, Standard Oil aggressively (see the Cleveland Massacre) built an integrated network of pipelines and refineries with standardized processes. As oil emerged as the dominant industrial resource, Standard Oil became dominant.
An Oil Baron vs. Data Baron
Instead of focusing on creating new data sources (eg. deploying industrial sensors or new systems of record) for more than a decade under Alex Karp's leadership Palantir aggressively sent Forward Deployed Engineers (FDEs) to customer sites. Using in house-tools like Magritte for ingestion, Contour for visualization, and Workshop for web applications, these FDEs would do the dirty work of data integration. By being on-site FDEs could navigate organizational dynamics, negotiate access permissions in person, and work through the thorny politics of data sharing that would have been impossible to resolve through remote meetings or formal requirements gathering (read more on this from Nabeel Qureshi). As data emerged as the dominant resource for unlocking AI, Palantir broke out as the dominant player by controlling the refinement and utilization of data for government and enterprises.
Foundry, Palantir's modern data refinery, now drives over 50% of revenue with 80% gross margins. And just as the discovery of new oil uses (automobiles, plastics, chemicals) massively increased the value of Standard Oil's refinery infrastructure in the early 1900s, the emergence of powerful AI models has dramatically increased the value of Palantir's data infrastructure in 2024. The market's dramatic revaluation reflects this shift from enterprise software company to the controller of many of the most valuable data gushers across enterprise and government.
Horizontal Challenges for Data Gushers
As Pete Mathias (Partner at Alumni Ventures) said in his investing ideas for 2025, there is “Trillion-Dollar Built World Goldmine” yet to be tapped → “If 70% of the economy is services and ‘services as software’ is tapped out, the next frontier = 30% built world. You can’t just “AI-ify” physical systems — you need sensors, IoT, and infra for operational data, etc. An edge compute behemoth exceeding today’s Cloud Giants combined is coming.”
However, to unlock this opportunity requires companies to solve a set of problems that exist across verticals (from manufacturing to mining). Below are the three we think are most important:
1/ Edge Processing → whether we want to track every high value package individually or monitor a fleet of remote mining assets, we need to develop low-downtime, energy efficient systems for capturing, analyzing, and networking the data. Edge processing companies today are benefitting from the efforts of startups like Swarm Technologies (acquired by SpaceX), that took significant risk (including being fined by the FCC for deploying their satellites without approval due to the risk of them becoming invisible space debris due to their size) to solve the frontier networking problem. One opportunity we are particularly interested in today is the development of novel chips with custom networking for direct to satellite connectivity and indoor operations.
2/ Privacy → if we hope to gather data at the edge (aka everywhere we work and live), then we will need to decide how to protect the privacy of individuals that still allow for new models to be developed using said data. One path to this is the continued investment and development of homomorphic encryption techniques which allows computations to be performed on encrypted data without first having to decrypt it. Numerai helped pioneer the application of this approach by developing an on-chain tournament where data-scientists could compete to predict the stock market using proprietary data without ever decrypting the data.
3/ Capability-Reliability Gap → many AI capabilities exist but are unreliable in high-stakes industrial contexts. Startups bridging this gap can unlock massive value. Aurora Innovation ($12B market cap public company founded in 2017) focused on reliability in self-driving technology for freight logistics, targeting a specific and profitable niche.
Ultimately, we believe that these challenges will be solved. The true rate limiter, as always, on capturing the value of data gushers is ambitious entrepreneurs. As Arvind Narayanan, Benedikt Ströbl, and Sayash Kapoor stated in their recent piece Is AI Progress Slowing Down, “the connection between capability improvements and AI’s social or economic impacts is extremely weak. The bottlenecks for impact are the pace of product development and the rate of adoption, not AI capabilities.”
If we don't succeed at solving these horizontal challenges or developing new sensors to unlock more data gushers, we will be pushed to develop synthetic data solutions (models that make data for other models to learn from). Funnily enough, this is the path that the oil industry is following. Oil is finite, but companies like Valar Atomics are unlocking infinite scale by synthesizing long-chain hydrocarbons from water and sequestered carbon using nuclear reactors (with others pursuing adjacent opportunities via hydrogen electrolysis like Terraform Industries, Rivan, and General Galactic). We look forward to an abundant future of (carbon-neutral) hydro-carbons and data.